NumPy: how to left join arrays with duplicates
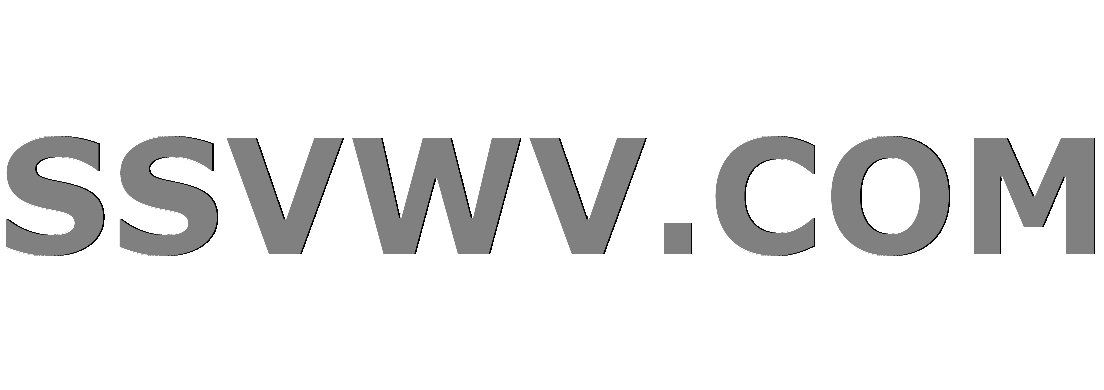
Multi tool use
To use Cython, I need to convert df1.merge(df2, how='left')
(using Pandas
) to plain NumPy
, while I found numpy.lib.recfunctions.join_by(key, r1, r2, jointype='leftouter')
doesn't support any duplicates along key
. Is there any way to solve it?
python pandas numpy cython
add a comment |
To use Cython, I need to convert df1.merge(df2, how='left')
(using Pandas
) to plain NumPy
, while I found numpy.lib.recfunctions.join_by(key, r1, r2, jointype='leftouter')
doesn't support any duplicates along key
. Is there any way to solve it?
python pandas numpy cython
2
The basic idea in mostrecfunctions
is to define a new dtype, create the appropriate 'empty' array, and copy values by field name. It's all readable python; no hidden compiled code. If existing functions don't do the job (they aren't heavily used or tested), write your own.
– hpaulj
Nov 12 '18 at 8:03
add a comment |
To use Cython, I need to convert df1.merge(df2, how='left')
(using Pandas
) to plain NumPy
, while I found numpy.lib.recfunctions.join_by(key, r1, r2, jointype='leftouter')
doesn't support any duplicates along key
. Is there any way to solve it?
python pandas numpy cython
To use Cython, I need to convert df1.merge(df2, how='left')
(using Pandas
) to plain NumPy
, while I found numpy.lib.recfunctions.join_by(key, r1, r2, jointype='leftouter')
doesn't support any duplicates along key
. Is there any way to solve it?
python pandas numpy cython
python pandas numpy cython
edited Nov 12 '18 at 8:03
betontalpfa
8451023
8451023
asked Nov 12 '18 at 7:58
NaiveNaive
80211
80211
2
The basic idea in mostrecfunctions
is to define a new dtype, create the appropriate 'empty' array, and copy values by field name. It's all readable python; no hidden compiled code. If existing functions don't do the job (they aren't heavily used or tested), write your own.
– hpaulj
Nov 12 '18 at 8:03
add a comment |
2
The basic idea in mostrecfunctions
is to define a new dtype, create the appropriate 'empty' array, and copy values by field name. It's all readable python; no hidden compiled code. If existing functions don't do the job (they aren't heavily used or tested), write your own.
– hpaulj
Nov 12 '18 at 8:03
2
2
The basic idea in most
recfunctions
is to define a new dtype, create the appropriate 'empty' array, and copy values by field name. It's all readable python; no hidden compiled code. If existing functions don't do the job (they aren't heavily used or tested), write your own.– hpaulj
Nov 12 '18 at 8:03
The basic idea in most
recfunctions
is to define a new dtype, create the appropriate 'empty' array, and copy values by field name. It's all readable python; no hidden compiled code. If existing functions don't do the job (they aren't heavily used or tested), write your own.– hpaulj
Nov 12 '18 at 8:03
add a comment |
1 Answer
1
active
oldest
votes
Here's a stab at a pure numpy
left join that can handle duplicate keys:
import numpy as np
def join_by_left(key, r1, r2, mask=True):
# figure out the dtype of the result array
descr1 = r1.dtype.descr
descr2 = [d for d in r2.dtype.descr if d[0] not in r1.dtype.names]
descrm = descr1 + descr2
# figure out the fields we'll need from each array
f1 = [d[0] for d in descr1]
f2 = [d[0] for d in descr2]
# cache the number of columns in f1
ncol1 = len(f1)
# get a dict of the rows of r2 grouped by key
rows2 =
for row2 in r2:
rows2.setdefault(row2[key], ).append(row2)
# figure out how many rows will be in the result
nrowm = 0
for k1 in r1[key]:
if k1 in rows2:
nrowm += len(rows2[k1])
else:
nrowm += 1
# allocate the return array
_ret = np.recarray(nrowm, dtype=descrm)
if mask:
ret = np.ma.array(_ret, mask=True)
else:
ret = _ret
# merge the data into the return array
i = 0
for row1 in r1:
if row1[key] in rows2:
for row2 in rows2[row1[key]]:
ret[i] = tuple(row1[f1]) + tuple(row2[f2])
i += 1
else:
for j in range(ncol1):
ret[i][j] = row1[j]
i += 1
return ret
Basically, it uses a plain dict
to do the actual join operation. Like numpy.lib.recfunctions.join_by
, this func will also return a masked array. When there are keys missing from the right array, those values will be masked out in the return array. If you would prefer a record array instead (in which all of the missing data is set to 0), you can just pass mask=False
when calling join_by_left
.
add a comment |
Your Answer
StackExchange.ifUsing("editor", function ()
StackExchange.using("externalEditor", function ()
StackExchange.using("snippets", function ()
StackExchange.snippets.init();
);
);
, "code-snippets");
StackExchange.ready(function()
var channelOptions =
tags: "".split(" "),
id: "1"
;
initTagRenderer("".split(" "), "".split(" "), channelOptions);
StackExchange.using("externalEditor", function()
// Have to fire editor after snippets, if snippets enabled
if (StackExchange.settings.snippets.snippetsEnabled)
StackExchange.using("snippets", function()
createEditor();
);
else
createEditor();
);
function createEditor()
StackExchange.prepareEditor(
heartbeatType: 'answer',
autoActivateHeartbeat: false,
convertImagesToLinks: true,
noModals: true,
showLowRepImageUploadWarning: true,
reputationToPostImages: 10,
bindNavPrevention: true,
postfix: "",
imageUploader:
brandingHtml: "Powered by u003ca class="icon-imgur-white" href="https://imgur.com/"u003eu003c/au003e",
contentPolicyHtml: "User contributions licensed under u003ca href="https://creativecommons.org/licenses/by-sa/3.0/"u003ecc by-sa 3.0 with attribution requiredu003c/au003e u003ca href="https://stackoverflow.com/legal/content-policy"u003e(content policy)u003c/au003e",
allowUrls: true
,
onDemand: true,
discardSelector: ".discard-answer"
,immediatelyShowMarkdownHelp:true
);
);
Sign up or log in
StackExchange.ready(function ()
StackExchange.helpers.onClickDraftSave('#login-link');
);
Sign up using Google
Sign up using Facebook
Sign up using Email and Password
Post as a guest
Required, but never shown
StackExchange.ready(
function ()
StackExchange.openid.initPostLogin('.new-post-login', 'https%3a%2f%2fstackoverflow.com%2fquestions%2f53257916%2fnumpy-how-to-left-join-arrays-with-duplicates%23new-answer', 'question_page');
);
Post as a guest
Required, but never shown
1 Answer
1
active
oldest
votes
1 Answer
1
active
oldest
votes
active
oldest
votes
active
oldest
votes
Here's a stab at a pure numpy
left join that can handle duplicate keys:
import numpy as np
def join_by_left(key, r1, r2, mask=True):
# figure out the dtype of the result array
descr1 = r1.dtype.descr
descr2 = [d for d in r2.dtype.descr if d[0] not in r1.dtype.names]
descrm = descr1 + descr2
# figure out the fields we'll need from each array
f1 = [d[0] for d in descr1]
f2 = [d[0] for d in descr2]
# cache the number of columns in f1
ncol1 = len(f1)
# get a dict of the rows of r2 grouped by key
rows2 =
for row2 in r2:
rows2.setdefault(row2[key], ).append(row2)
# figure out how many rows will be in the result
nrowm = 0
for k1 in r1[key]:
if k1 in rows2:
nrowm += len(rows2[k1])
else:
nrowm += 1
# allocate the return array
_ret = np.recarray(nrowm, dtype=descrm)
if mask:
ret = np.ma.array(_ret, mask=True)
else:
ret = _ret
# merge the data into the return array
i = 0
for row1 in r1:
if row1[key] in rows2:
for row2 in rows2[row1[key]]:
ret[i] = tuple(row1[f1]) + tuple(row2[f2])
i += 1
else:
for j in range(ncol1):
ret[i][j] = row1[j]
i += 1
return ret
Basically, it uses a plain dict
to do the actual join operation. Like numpy.lib.recfunctions.join_by
, this func will also return a masked array. When there are keys missing from the right array, those values will be masked out in the return array. If you would prefer a record array instead (in which all of the missing data is set to 0), you can just pass mask=False
when calling join_by_left
.
add a comment |
Here's a stab at a pure numpy
left join that can handle duplicate keys:
import numpy as np
def join_by_left(key, r1, r2, mask=True):
# figure out the dtype of the result array
descr1 = r1.dtype.descr
descr2 = [d for d in r2.dtype.descr if d[0] not in r1.dtype.names]
descrm = descr1 + descr2
# figure out the fields we'll need from each array
f1 = [d[0] for d in descr1]
f2 = [d[0] for d in descr2]
# cache the number of columns in f1
ncol1 = len(f1)
# get a dict of the rows of r2 grouped by key
rows2 =
for row2 in r2:
rows2.setdefault(row2[key], ).append(row2)
# figure out how many rows will be in the result
nrowm = 0
for k1 in r1[key]:
if k1 in rows2:
nrowm += len(rows2[k1])
else:
nrowm += 1
# allocate the return array
_ret = np.recarray(nrowm, dtype=descrm)
if mask:
ret = np.ma.array(_ret, mask=True)
else:
ret = _ret
# merge the data into the return array
i = 0
for row1 in r1:
if row1[key] in rows2:
for row2 in rows2[row1[key]]:
ret[i] = tuple(row1[f1]) + tuple(row2[f2])
i += 1
else:
for j in range(ncol1):
ret[i][j] = row1[j]
i += 1
return ret
Basically, it uses a plain dict
to do the actual join operation. Like numpy.lib.recfunctions.join_by
, this func will also return a masked array. When there are keys missing from the right array, those values will be masked out in the return array. If you would prefer a record array instead (in which all of the missing data is set to 0), you can just pass mask=False
when calling join_by_left
.
add a comment |
Here's a stab at a pure numpy
left join that can handle duplicate keys:
import numpy as np
def join_by_left(key, r1, r2, mask=True):
# figure out the dtype of the result array
descr1 = r1.dtype.descr
descr2 = [d for d in r2.dtype.descr if d[0] not in r1.dtype.names]
descrm = descr1 + descr2
# figure out the fields we'll need from each array
f1 = [d[0] for d in descr1]
f2 = [d[0] for d in descr2]
# cache the number of columns in f1
ncol1 = len(f1)
# get a dict of the rows of r2 grouped by key
rows2 =
for row2 in r2:
rows2.setdefault(row2[key], ).append(row2)
# figure out how many rows will be in the result
nrowm = 0
for k1 in r1[key]:
if k1 in rows2:
nrowm += len(rows2[k1])
else:
nrowm += 1
# allocate the return array
_ret = np.recarray(nrowm, dtype=descrm)
if mask:
ret = np.ma.array(_ret, mask=True)
else:
ret = _ret
# merge the data into the return array
i = 0
for row1 in r1:
if row1[key] in rows2:
for row2 in rows2[row1[key]]:
ret[i] = tuple(row1[f1]) + tuple(row2[f2])
i += 1
else:
for j in range(ncol1):
ret[i][j] = row1[j]
i += 1
return ret
Basically, it uses a plain dict
to do the actual join operation. Like numpy.lib.recfunctions.join_by
, this func will also return a masked array. When there are keys missing from the right array, those values will be masked out in the return array. If you would prefer a record array instead (in which all of the missing data is set to 0), you can just pass mask=False
when calling join_by_left
.
Here's a stab at a pure numpy
left join that can handle duplicate keys:
import numpy as np
def join_by_left(key, r1, r2, mask=True):
# figure out the dtype of the result array
descr1 = r1.dtype.descr
descr2 = [d for d in r2.dtype.descr if d[0] not in r1.dtype.names]
descrm = descr1 + descr2
# figure out the fields we'll need from each array
f1 = [d[0] for d in descr1]
f2 = [d[0] for d in descr2]
# cache the number of columns in f1
ncol1 = len(f1)
# get a dict of the rows of r2 grouped by key
rows2 =
for row2 in r2:
rows2.setdefault(row2[key], ).append(row2)
# figure out how many rows will be in the result
nrowm = 0
for k1 in r1[key]:
if k1 in rows2:
nrowm += len(rows2[k1])
else:
nrowm += 1
# allocate the return array
_ret = np.recarray(nrowm, dtype=descrm)
if mask:
ret = np.ma.array(_ret, mask=True)
else:
ret = _ret
# merge the data into the return array
i = 0
for row1 in r1:
if row1[key] in rows2:
for row2 in rows2[row1[key]]:
ret[i] = tuple(row1[f1]) + tuple(row2[f2])
i += 1
else:
for j in range(ncol1):
ret[i][j] = row1[j]
i += 1
return ret
Basically, it uses a plain dict
to do the actual join operation. Like numpy.lib.recfunctions.join_by
, this func will also return a masked array. When there are keys missing from the right array, those values will be masked out in the return array. If you would prefer a record array instead (in which all of the missing data is set to 0), you can just pass mask=False
when calling join_by_left
.
edited Nov 12 '18 at 12:52
answered Nov 12 '18 at 12:07


teltel
6,19321430
6,19321430
add a comment |
add a comment |
Thanks for contributing an answer to Stack Overflow!
- Please be sure to answer the question. Provide details and share your research!
But avoid …
- Asking for help, clarification, or responding to other answers.
- Making statements based on opinion; back them up with references or personal experience.
To learn more, see our tips on writing great answers.
Sign up or log in
StackExchange.ready(function ()
StackExchange.helpers.onClickDraftSave('#login-link');
);
Sign up using Google
Sign up using Facebook
Sign up using Email and Password
Post as a guest
Required, but never shown
StackExchange.ready(
function ()
StackExchange.openid.initPostLogin('.new-post-login', 'https%3a%2f%2fstackoverflow.com%2fquestions%2f53257916%2fnumpy-how-to-left-join-arrays-with-duplicates%23new-answer', 'question_page');
);
Post as a guest
Required, but never shown
Sign up or log in
StackExchange.ready(function ()
StackExchange.helpers.onClickDraftSave('#login-link');
);
Sign up using Google
Sign up using Facebook
Sign up using Email and Password
Post as a guest
Required, but never shown
Sign up or log in
StackExchange.ready(function ()
StackExchange.helpers.onClickDraftSave('#login-link');
);
Sign up using Google
Sign up using Facebook
Sign up using Email and Password
Post as a guest
Required, but never shown
Sign up or log in
StackExchange.ready(function ()
StackExchange.helpers.onClickDraftSave('#login-link');
);
Sign up using Google
Sign up using Facebook
Sign up using Email and Password
Sign up using Google
Sign up using Facebook
Sign up using Email and Password
Post as a guest
Required, but never shown
Required, but never shown
Required, but never shown
Required, but never shown
Required, but never shown
Required, but never shown
Required, but never shown
Required, but never shown
Required, but never shown
bRfa,Us yMzBq
2
The basic idea in most
recfunctions
is to define a new dtype, create the appropriate 'empty' array, and copy values by field name. It's all readable python; no hidden compiled code. If existing functions don't do the job (they aren't heavily used or tested), write your own.– hpaulj
Nov 12 '18 at 8:03