return the top_k masked softmax of each row for a 2D tensor
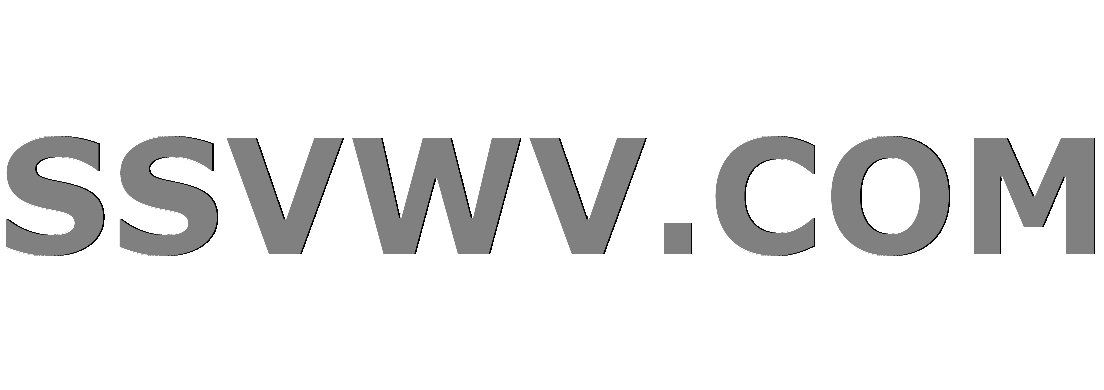
Multi tool use
For any 2D tensor like
[[2,5,4,7],
[7,5,6,8]],
I want to do softmax for the top k element in each row and then construct a new tensor by replacing all the other elements to 0.
The result should be to get the softmax of top k (here k=2) elements for each row [[7,5],[8,7]],
which is thus
[[0.880797,0.11920291],
[0.7310586,0.26894143]]
and then reconstruct a new tensor according to the index of the top k elements in the original tensor, the final result should be
[[0,0.11920291,0,0.880797],
[0.26894143,0,0,0.7310586]].
Is it possible to implement this kind of masked softmax in tensorflow? Many thanks in advance!
python tensorflow indexing tensor softmax
add a comment |
For any 2D tensor like
[[2,5,4,7],
[7,5,6,8]],
I want to do softmax for the top k element in each row and then construct a new tensor by replacing all the other elements to 0.
The result should be to get the softmax of top k (here k=2) elements for each row [[7,5],[8,7]],
which is thus
[[0.880797,0.11920291],
[0.7310586,0.26894143]]
and then reconstruct a new tensor according to the index of the top k elements in the original tensor, the final result should be
[[0,0.11920291,0,0.880797],
[0.26894143,0,0,0.7310586]].
Is it possible to implement this kind of masked softmax in tensorflow? Many thanks in advance!
python tensorflow indexing tensor softmax
add a comment |
For any 2D tensor like
[[2,5,4,7],
[7,5,6,8]],
I want to do softmax for the top k element in each row and then construct a new tensor by replacing all the other elements to 0.
The result should be to get the softmax of top k (here k=2) elements for each row [[7,5],[8,7]],
which is thus
[[0.880797,0.11920291],
[0.7310586,0.26894143]]
and then reconstruct a new tensor according to the index of the top k elements in the original tensor, the final result should be
[[0,0.11920291,0,0.880797],
[0.26894143,0,0,0.7310586]].
Is it possible to implement this kind of masked softmax in tensorflow? Many thanks in advance!
python tensorflow indexing tensor softmax
For any 2D tensor like
[[2,5,4,7],
[7,5,6,8]],
I want to do softmax for the top k element in each row and then construct a new tensor by replacing all the other elements to 0.
The result should be to get the softmax of top k (here k=2) elements for each row [[7,5],[8,7]],
which is thus
[[0.880797,0.11920291],
[0.7310586,0.26894143]]
and then reconstruct a new tensor according to the index of the top k elements in the original tensor, the final result should be
[[0,0.11920291,0,0.880797],
[0.26894143,0,0,0.7310586]].
Is it possible to implement this kind of masked softmax in tensorflow? Many thanks in advance!
python tensorflow indexing tensor softmax
python tensorflow indexing tensor softmax
asked Nov 13 '18 at 12:20
clement116clement116
656
656
add a comment |
add a comment |
1 Answer
1
active
oldest
votes
Here is how you can do that:
import tensorflow as tf
# Input data
a = tf.placeholder(tf.float32, [None, None])
num_top = tf.placeholder(tf.int32, )
# Find top elements
a_top, a_top_idx = tf.nn.top_k(a, num_top, sorted=False)
# Apply softmax
a_top_sm = tf.nn.softmax(a_top)
# Reconstruct into original shape
a_shape = tf.shape(a)
a_row_idx = tf.tile(tf.range(a_shape[0])[:, tf.newaxis], (1, num_top))
scatter_idx = tf.stack([a_row_idx, a_top_idx], axis=-1)
result = tf.scatter_nd(scatter_idx, a_top_sm, a_shape)
# Test
with tf.Session() as sess:
result_val = sess.run(result, feed_dict=a: [[2, 5, 4, 7], [7, 5, 6, 8]], num_top: 2)
print(result_val)
Output:
[[0. 0.11920291 0. 0.880797 ]
[0.26894143 0. 0. 0.7310586 ]]
EDIT:
Actually, there is a function that more closely does what you intend, tf.sparse.softmax
. However, it requires a SparseTensor
as input, and I'm not sure it should be faster since it has to figure out which sparse values go together in the softmax. The good thing about this function is that you could have different number of elements to softmax in each row, but in your case that does not seem to be important. Anyway, here is an implementation with that, in case you find it useful.
import tensorflow as tf
a = tf.placeholder(tf.float32, [None, None])
num_top = tf.placeholder(tf.int32, )
# Find top elements
a_top, a_top_idx = tf.nn.top_k(a, num_top, sorted=False)
# Flatten values
sparse_values = tf.reshape(a_top, [-1])
# Make sparse indices
shape = tf.cast(tf.shape(a), tf.int64)
a_row_idx = tf.tile(tf.range(shape[0])[:, tf.newaxis], (1, num_top))
sparse_idx = tf.stack([a_row_idx, tf.cast(a_top_idx, tf.int64)], axis=-1)
sparse_idx = tf.reshape(sparse_idx, [-1, 2])
# Make sparse tensor
a_top_sparse = tf.SparseTensor(sparse_idx, sparse_values, shape)
# Reorder sparse tensor
a_top_sparse = tf.sparse.reorder(a_top_sparse)
# Softmax
result_sparse = tf.sparse.softmax(a_top_sparse)
# Convert back to dense (or you can keep working with the sparse tensor)
result = tf.sparse.to_dense(result_sparse)
# Test
with tf.Session() as sess:
result_val = sess.run(result, feed_dict=a: [[2, 5, 4, 7], [7, 5, 6, 8]], num_top: 2)
print(result_val)
# Same as before
Thank you a lot @jdehesa! For the sparse_softmax part, I find that I had to change the line to "result = tf.sparse_tensor_to_dense(result_sparse,validate_indices=False)" to run the code without error. However, the non-zero elements in each row are ranked descendently, like this [[0. 0.880797 0. 0.11920291] [0.7310586 0. 0. 0.26894143]]. It seems the tf.sparse_softmax will automatically rank the element decendently. Is it possible to solve this?
– clement116
Nov 14 '18 at 0:52
The first program looks really cool, especially the use of tf.tile, tf.stack and tf.scatter_nd. Learned a lot, thanks.
– clement116
Nov 14 '18 at 1:14
Hi @jdehesa, I solved this problem. We just need to reorder the indexes of the a_top_sparse before put it into tf.sparse_softmax. This is done by a_top_sparse = tf.sparse_reorder(a_top_sparse)
– clement116
Nov 14 '18 at 7:29
@clement116 That's interesting, it seems to work fine for me without it (v1.12.0), but looking at the implementation oftf.sparse.softmax
andtf.sparse.to_dense
it seems the operations do assume that the sparse tensor is ordered (I think). Thanks for finding that out, I updated the answer.
– jdehesa
Nov 14 '18 at 9:51
ah, I use v1.8.0, that's the problem.
– clement116
Nov 14 '18 at 12:35
add a comment |
Your Answer
StackExchange.ifUsing("editor", function ()
StackExchange.using("externalEditor", function ()
StackExchange.using("snippets", function ()
StackExchange.snippets.init();
);
);
, "code-snippets");
StackExchange.ready(function()
var channelOptions =
tags: "".split(" "),
id: "1"
;
initTagRenderer("".split(" "), "".split(" "), channelOptions);
StackExchange.using("externalEditor", function()
// Have to fire editor after snippets, if snippets enabled
if (StackExchange.settings.snippets.snippetsEnabled)
StackExchange.using("snippets", function()
createEditor();
);
else
createEditor();
);
function createEditor()
StackExchange.prepareEditor(
heartbeatType: 'answer',
autoActivateHeartbeat: false,
convertImagesToLinks: true,
noModals: true,
showLowRepImageUploadWarning: true,
reputationToPostImages: 10,
bindNavPrevention: true,
postfix: "",
imageUploader:
brandingHtml: "Powered by u003ca class="icon-imgur-white" href="https://imgur.com/"u003eu003c/au003e",
contentPolicyHtml: "User contributions licensed under u003ca href="https://creativecommons.org/licenses/by-sa/3.0/"u003ecc by-sa 3.0 with attribution requiredu003c/au003e u003ca href="https://stackoverflow.com/legal/content-policy"u003e(content policy)u003c/au003e",
allowUrls: true
,
onDemand: true,
discardSelector: ".discard-answer"
,immediatelyShowMarkdownHelp:true
);
);
Sign up or log in
StackExchange.ready(function ()
StackExchange.helpers.onClickDraftSave('#login-link');
);
Sign up using Google
Sign up using Facebook
Sign up using Email and Password
Post as a guest
Required, but never shown
StackExchange.ready(
function ()
StackExchange.openid.initPostLogin('.new-post-login', 'https%3a%2f%2fstackoverflow.com%2fquestions%2f53280894%2freturn-the-top-k-masked-softmax-of-each-row-for-a-2d-tensor%23new-answer', 'question_page');
);
Post as a guest
Required, but never shown
1 Answer
1
active
oldest
votes
1 Answer
1
active
oldest
votes
active
oldest
votes
active
oldest
votes
Here is how you can do that:
import tensorflow as tf
# Input data
a = tf.placeholder(tf.float32, [None, None])
num_top = tf.placeholder(tf.int32, )
# Find top elements
a_top, a_top_idx = tf.nn.top_k(a, num_top, sorted=False)
# Apply softmax
a_top_sm = tf.nn.softmax(a_top)
# Reconstruct into original shape
a_shape = tf.shape(a)
a_row_idx = tf.tile(tf.range(a_shape[0])[:, tf.newaxis], (1, num_top))
scatter_idx = tf.stack([a_row_idx, a_top_idx], axis=-1)
result = tf.scatter_nd(scatter_idx, a_top_sm, a_shape)
# Test
with tf.Session() as sess:
result_val = sess.run(result, feed_dict=a: [[2, 5, 4, 7], [7, 5, 6, 8]], num_top: 2)
print(result_val)
Output:
[[0. 0.11920291 0. 0.880797 ]
[0.26894143 0. 0. 0.7310586 ]]
EDIT:
Actually, there is a function that more closely does what you intend, tf.sparse.softmax
. However, it requires a SparseTensor
as input, and I'm not sure it should be faster since it has to figure out which sparse values go together in the softmax. The good thing about this function is that you could have different number of elements to softmax in each row, but in your case that does not seem to be important. Anyway, here is an implementation with that, in case you find it useful.
import tensorflow as tf
a = tf.placeholder(tf.float32, [None, None])
num_top = tf.placeholder(tf.int32, )
# Find top elements
a_top, a_top_idx = tf.nn.top_k(a, num_top, sorted=False)
# Flatten values
sparse_values = tf.reshape(a_top, [-1])
# Make sparse indices
shape = tf.cast(tf.shape(a), tf.int64)
a_row_idx = tf.tile(tf.range(shape[0])[:, tf.newaxis], (1, num_top))
sparse_idx = tf.stack([a_row_idx, tf.cast(a_top_idx, tf.int64)], axis=-1)
sparse_idx = tf.reshape(sparse_idx, [-1, 2])
# Make sparse tensor
a_top_sparse = tf.SparseTensor(sparse_idx, sparse_values, shape)
# Reorder sparse tensor
a_top_sparse = tf.sparse.reorder(a_top_sparse)
# Softmax
result_sparse = tf.sparse.softmax(a_top_sparse)
# Convert back to dense (or you can keep working with the sparse tensor)
result = tf.sparse.to_dense(result_sparse)
# Test
with tf.Session() as sess:
result_val = sess.run(result, feed_dict=a: [[2, 5, 4, 7], [7, 5, 6, 8]], num_top: 2)
print(result_val)
# Same as before
Thank you a lot @jdehesa! For the sparse_softmax part, I find that I had to change the line to "result = tf.sparse_tensor_to_dense(result_sparse,validate_indices=False)" to run the code without error. However, the non-zero elements in each row are ranked descendently, like this [[0. 0.880797 0. 0.11920291] [0.7310586 0. 0. 0.26894143]]. It seems the tf.sparse_softmax will automatically rank the element decendently. Is it possible to solve this?
– clement116
Nov 14 '18 at 0:52
The first program looks really cool, especially the use of tf.tile, tf.stack and tf.scatter_nd. Learned a lot, thanks.
– clement116
Nov 14 '18 at 1:14
Hi @jdehesa, I solved this problem. We just need to reorder the indexes of the a_top_sparse before put it into tf.sparse_softmax. This is done by a_top_sparse = tf.sparse_reorder(a_top_sparse)
– clement116
Nov 14 '18 at 7:29
@clement116 That's interesting, it seems to work fine for me without it (v1.12.0), but looking at the implementation oftf.sparse.softmax
andtf.sparse.to_dense
it seems the operations do assume that the sparse tensor is ordered (I think). Thanks for finding that out, I updated the answer.
– jdehesa
Nov 14 '18 at 9:51
ah, I use v1.8.0, that's the problem.
– clement116
Nov 14 '18 at 12:35
add a comment |
Here is how you can do that:
import tensorflow as tf
# Input data
a = tf.placeholder(tf.float32, [None, None])
num_top = tf.placeholder(tf.int32, )
# Find top elements
a_top, a_top_idx = tf.nn.top_k(a, num_top, sorted=False)
# Apply softmax
a_top_sm = tf.nn.softmax(a_top)
# Reconstruct into original shape
a_shape = tf.shape(a)
a_row_idx = tf.tile(tf.range(a_shape[0])[:, tf.newaxis], (1, num_top))
scatter_idx = tf.stack([a_row_idx, a_top_idx], axis=-1)
result = tf.scatter_nd(scatter_idx, a_top_sm, a_shape)
# Test
with tf.Session() as sess:
result_val = sess.run(result, feed_dict=a: [[2, 5, 4, 7], [7, 5, 6, 8]], num_top: 2)
print(result_val)
Output:
[[0. 0.11920291 0. 0.880797 ]
[0.26894143 0. 0. 0.7310586 ]]
EDIT:
Actually, there is a function that more closely does what you intend, tf.sparse.softmax
. However, it requires a SparseTensor
as input, and I'm not sure it should be faster since it has to figure out which sparse values go together in the softmax. The good thing about this function is that you could have different number of elements to softmax in each row, but in your case that does not seem to be important. Anyway, here is an implementation with that, in case you find it useful.
import tensorflow as tf
a = tf.placeholder(tf.float32, [None, None])
num_top = tf.placeholder(tf.int32, )
# Find top elements
a_top, a_top_idx = tf.nn.top_k(a, num_top, sorted=False)
# Flatten values
sparse_values = tf.reshape(a_top, [-1])
# Make sparse indices
shape = tf.cast(tf.shape(a), tf.int64)
a_row_idx = tf.tile(tf.range(shape[0])[:, tf.newaxis], (1, num_top))
sparse_idx = tf.stack([a_row_idx, tf.cast(a_top_idx, tf.int64)], axis=-1)
sparse_idx = tf.reshape(sparse_idx, [-1, 2])
# Make sparse tensor
a_top_sparse = tf.SparseTensor(sparse_idx, sparse_values, shape)
# Reorder sparse tensor
a_top_sparse = tf.sparse.reorder(a_top_sparse)
# Softmax
result_sparse = tf.sparse.softmax(a_top_sparse)
# Convert back to dense (or you can keep working with the sparse tensor)
result = tf.sparse.to_dense(result_sparse)
# Test
with tf.Session() as sess:
result_val = sess.run(result, feed_dict=a: [[2, 5, 4, 7], [7, 5, 6, 8]], num_top: 2)
print(result_val)
# Same as before
Thank you a lot @jdehesa! For the sparse_softmax part, I find that I had to change the line to "result = tf.sparse_tensor_to_dense(result_sparse,validate_indices=False)" to run the code without error. However, the non-zero elements in each row are ranked descendently, like this [[0. 0.880797 0. 0.11920291] [0.7310586 0. 0. 0.26894143]]. It seems the tf.sparse_softmax will automatically rank the element decendently. Is it possible to solve this?
– clement116
Nov 14 '18 at 0:52
The first program looks really cool, especially the use of tf.tile, tf.stack and tf.scatter_nd. Learned a lot, thanks.
– clement116
Nov 14 '18 at 1:14
Hi @jdehesa, I solved this problem. We just need to reorder the indexes of the a_top_sparse before put it into tf.sparse_softmax. This is done by a_top_sparse = tf.sparse_reorder(a_top_sparse)
– clement116
Nov 14 '18 at 7:29
@clement116 That's interesting, it seems to work fine for me without it (v1.12.0), but looking at the implementation oftf.sparse.softmax
andtf.sparse.to_dense
it seems the operations do assume that the sparse tensor is ordered (I think). Thanks for finding that out, I updated the answer.
– jdehesa
Nov 14 '18 at 9:51
ah, I use v1.8.0, that's the problem.
– clement116
Nov 14 '18 at 12:35
add a comment |
Here is how you can do that:
import tensorflow as tf
# Input data
a = tf.placeholder(tf.float32, [None, None])
num_top = tf.placeholder(tf.int32, )
# Find top elements
a_top, a_top_idx = tf.nn.top_k(a, num_top, sorted=False)
# Apply softmax
a_top_sm = tf.nn.softmax(a_top)
# Reconstruct into original shape
a_shape = tf.shape(a)
a_row_idx = tf.tile(tf.range(a_shape[0])[:, tf.newaxis], (1, num_top))
scatter_idx = tf.stack([a_row_idx, a_top_idx], axis=-1)
result = tf.scatter_nd(scatter_idx, a_top_sm, a_shape)
# Test
with tf.Session() as sess:
result_val = sess.run(result, feed_dict=a: [[2, 5, 4, 7], [7, 5, 6, 8]], num_top: 2)
print(result_val)
Output:
[[0. 0.11920291 0. 0.880797 ]
[0.26894143 0. 0. 0.7310586 ]]
EDIT:
Actually, there is a function that more closely does what you intend, tf.sparse.softmax
. However, it requires a SparseTensor
as input, and I'm not sure it should be faster since it has to figure out which sparse values go together in the softmax. The good thing about this function is that you could have different number of elements to softmax in each row, but in your case that does not seem to be important. Anyway, here is an implementation with that, in case you find it useful.
import tensorflow as tf
a = tf.placeholder(tf.float32, [None, None])
num_top = tf.placeholder(tf.int32, )
# Find top elements
a_top, a_top_idx = tf.nn.top_k(a, num_top, sorted=False)
# Flatten values
sparse_values = tf.reshape(a_top, [-1])
# Make sparse indices
shape = tf.cast(tf.shape(a), tf.int64)
a_row_idx = tf.tile(tf.range(shape[0])[:, tf.newaxis], (1, num_top))
sparse_idx = tf.stack([a_row_idx, tf.cast(a_top_idx, tf.int64)], axis=-1)
sparse_idx = tf.reshape(sparse_idx, [-1, 2])
# Make sparse tensor
a_top_sparse = tf.SparseTensor(sparse_idx, sparse_values, shape)
# Reorder sparse tensor
a_top_sparse = tf.sparse.reorder(a_top_sparse)
# Softmax
result_sparse = tf.sparse.softmax(a_top_sparse)
# Convert back to dense (or you can keep working with the sparse tensor)
result = tf.sparse.to_dense(result_sparse)
# Test
with tf.Session() as sess:
result_val = sess.run(result, feed_dict=a: [[2, 5, 4, 7], [7, 5, 6, 8]], num_top: 2)
print(result_val)
# Same as before
Here is how you can do that:
import tensorflow as tf
# Input data
a = tf.placeholder(tf.float32, [None, None])
num_top = tf.placeholder(tf.int32, )
# Find top elements
a_top, a_top_idx = tf.nn.top_k(a, num_top, sorted=False)
# Apply softmax
a_top_sm = tf.nn.softmax(a_top)
# Reconstruct into original shape
a_shape = tf.shape(a)
a_row_idx = tf.tile(tf.range(a_shape[0])[:, tf.newaxis], (1, num_top))
scatter_idx = tf.stack([a_row_idx, a_top_idx], axis=-1)
result = tf.scatter_nd(scatter_idx, a_top_sm, a_shape)
# Test
with tf.Session() as sess:
result_val = sess.run(result, feed_dict=a: [[2, 5, 4, 7], [7, 5, 6, 8]], num_top: 2)
print(result_val)
Output:
[[0. 0.11920291 0. 0.880797 ]
[0.26894143 0. 0. 0.7310586 ]]
EDIT:
Actually, there is a function that more closely does what you intend, tf.sparse.softmax
. However, it requires a SparseTensor
as input, and I'm not sure it should be faster since it has to figure out which sparse values go together in the softmax. The good thing about this function is that you could have different number of elements to softmax in each row, but in your case that does not seem to be important. Anyway, here is an implementation with that, in case you find it useful.
import tensorflow as tf
a = tf.placeholder(tf.float32, [None, None])
num_top = tf.placeholder(tf.int32, )
# Find top elements
a_top, a_top_idx = tf.nn.top_k(a, num_top, sorted=False)
# Flatten values
sparse_values = tf.reshape(a_top, [-1])
# Make sparse indices
shape = tf.cast(tf.shape(a), tf.int64)
a_row_idx = tf.tile(tf.range(shape[0])[:, tf.newaxis], (1, num_top))
sparse_idx = tf.stack([a_row_idx, tf.cast(a_top_idx, tf.int64)], axis=-1)
sparse_idx = tf.reshape(sparse_idx, [-1, 2])
# Make sparse tensor
a_top_sparse = tf.SparseTensor(sparse_idx, sparse_values, shape)
# Reorder sparse tensor
a_top_sparse = tf.sparse.reorder(a_top_sparse)
# Softmax
result_sparse = tf.sparse.softmax(a_top_sparse)
# Convert back to dense (or you can keep working with the sparse tensor)
result = tf.sparse.to_dense(result_sparse)
# Test
with tf.Session() as sess:
result_val = sess.run(result, feed_dict=a: [[2, 5, 4, 7], [7, 5, 6, 8]], num_top: 2)
print(result_val)
# Same as before
edited Nov 14 '18 at 9:51
answered Nov 13 '18 at 12:37
jdehesajdehesa
24k43554
24k43554
Thank you a lot @jdehesa! For the sparse_softmax part, I find that I had to change the line to "result = tf.sparse_tensor_to_dense(result_sparse,validate_indices=False)" to run the code without error. However, the non-zero elements in each row are ranked descendently, like this [[0. 0.880797 0. 0.11920291] [0.7310586 0. 0. 0.26894143]]. It seems the tf.sparse_softmax will automatically rank the element decendently. Is it possible to solve this?
– clement116
Nov 14 '18 at 0:52
The first program looks really cool, especially the use of tf.tile, tf.stack and tf.scatter_nd. Learned a lot, thanks.
– clement116
Nov 14 '18 at 1:14
Hi @jdehesa, I solved this problem. We just need to reorder the indexes of the a_top_sparse before put it into tf.sparse_softmax. This is done by a_top_sparse = tf.sparse_reorder(a_top_sparse)
– clement116
Nov 14 '18 at 7:29
@clement116 That's interesting, it seems to work fine for me without it (v1.12.0), but looking at the implementation oftf.sparse.softmax
andtf.sparse.to_dense
it seems the operations do assume that the sparse tensor is ordered (I think). Thanks for finding that out, I updated the answer.
– jdehesa
Nov 14 '18 at 9:51
ah, I use v1.8.0, that's the problem.
– clement116
Nov 14 '18 at 12:35
add a comment |
Thank you a lot @jdehesa! For the sparse_softmax part, I find that I had to change the line to "result = tf.sparse_tensor_to_dense(result_sparse,validate_indices=False)" to run the code without error. However, the non-zero elements in each row are ranked descendently, like this [[0. 0.880797 0. 0.11920291] [0.7310586 0. 0. 0.26894143]]. It seems the tf.sparse_softmax will automatically rank the element decendently. Is it possible to solve this?
– clement116
Nov 14 '18 at 0:52
The first program looks really cool, especially the use of tf.tile, tf.stack and tf.scatter_nd. Learned a lot, thanks.
– clement116
Nov 14 '18 at 1:14
Hi @jdehesa, I solved this problem. We just need to reorder the indexes of the a_top_sparse before put it into tf.sparse_softmax. This is done by a_top_sparse = tf.sparse_reorder(a_top_sparse)
– clement116
Nov 14 '18 at 7:29
@clement116 That's interesting, it seems to work fine for me without it (v1.12.0), but looking at the implementation oftf.sparse.softmax
andtf.sparse.to_dense
it seems the operations do assume that the sparse tensor is ordered (I think). Thanks for finding that out, I updated the answer.
– jdehesa
Nov 14 '18 at 9:51
ah, I use v1.8.0, that's the problem.
– clement116
Nov 14 '18 at 12:35
Thank you a lot @jdehesa! For the sparse_softmax part, I find that I had to change the line to "result = tf.sparse_tensor_to_dense(result_sparse,validate_indices=False)" to run the code without error. However, the non-zero elements in each row are ranked descendently, like this [[0. 0.880797 0. 0.11920291] [0.7310586 0. 0. 0.26894143]]. It seems the tf.sparse_softmax will automatically rank the element decendently. Is it possible to solve this?
– clement116
Nov 14 '18 at 0:52
Thank you a lot @jdehesa! For the sparse_softmax part, I find that I had to change the line to "result = tf.sparse_tensor_to_dense(result_sparse,validate_indices=False)" to run the code without error. However, the non-zero elements in each row are ranked descendently, like this [[0. 0.880797 0. 0.11920291] [0.7310586 0. 0. 0.26894143]]. It seems the tf.sparse_softmax will automatically rank the element decendently. Is it possible to solve this?
– clement116
Nov 14 '18 at 0:52
The first program looks really cool, especially the use of tf.tile, tf.stack and tf.scatter_nd. Learned a lot, thanks.
– clement116
Nov 14 '18 at 1:14
The first program looks really cool, especially the use of tf.tile, tf.stack and tf.scatter_nd. Learned a lot, thanks.
– clement116
Nov 14 '18 at 1:14
Hi @jdehesa, I solved this problem. We just need to reorder the indexes of the a_top_sparse before put it into tf.sparse_softmax. This is done by a_top_sparse = tf.sparse_reorder(a_top_sparse)
– clement116
Nov 14 '18 at 7:29
Hi @jdehesa, I solved this problem. We just need to reorder the indexes of the a_top_sparse before put it into tf.sparse_softmax. This is done by a_top_sparse = tf.sparse_reorder(a_top_sparse)
– clement116
Nov 14 '18 at 7:29
@clement116 That's interesting, it seems to work fine for me without it (v1.12.0), but looking at the implementation of
tf.sparse.softmax
and tf.sparse.to_dense
it seems the operations do assume that the sparse tensor is ordered (I think). Thanks for finding that out, I updated the answer.– jdehesa
Nov 14 '18 at 9:51
@clement116 That's interesting, it seems to work fine for me without it (v1.12.0), but looking at the implementation of
tf.sparse.softmax
and tf.sparse.to_dense
it seems the operations do assume that the sparse tensor is ordered (I think). Thanks for finding that out, I updated the answer.– jdehesa
Nov 14 '18 at 9:51
ah, I use v1.8.0, that's the problem.
– clement116
Nov 14 '18 at 12:35
ah, I use v1.8.0, that's the problem.
– clement116
Nov 14 '18 at 12:35
add a comment |
Thanks for contributing an answer to Stack Overflow!
- Please be sure to answer the question. Provide details and share your research!
But avoid …
- Asking for help, clarification, or responding to other answers.
- Making statements based on opinion; back them up with references or personal experience.
To learn more, see our tips on writing great answers.
Sign up or log in
StackExchange.ready(function ()
StackExchange.helpers.onClickDraftSave('#login-link');
);
Sign up using Google
Sign up using Facebook
Sign up using Email and Password
Post as a guest
Required, but never shown
StackExchange.ready(
function ()
StackExchange.openid.initPostLogin('.new-post-login', 'https%3a%2f%2fstackoverflow.com%2fquestions%2f53280894%2freturn-the-top-k-masked-softmax-of-each-row-for-a-2d-tensor%23new-answer', 'question_page');
);
Post as a guest
Required, but never shown
Sign up or log in
StackExchange.ready(function ()
StackExchange.helpers.onClickDraftSave('#login-link');
);
Sign up using Google
Sign up using Facebook
Sign up using Email and Password
Post as a guest
Required, but never shown
Sign up or log in
StackExchange.ready(function ()
StackExchange.helpers.onClickDraftSave('#login-link');
);
Sign up using Google
Sign up using Facebook
Sign up using Email and Password
Post as a guest
Required, but never shown
Sign up or log in
StackExchange.ready(function ()
StackExchange.helpers.onClickDraftSave('#login-link');
);
Sign up using Google
Sign up using Facebook
Sign up using Email and Password
Sign up using Google
Sign up using Facebook
Sign up using Email and Password
Post as a guest
Required, but never shown
Required, but never shown
Required, but never shown
Required, but never shown
Required, but never shown
Required, but never shown
Required, but never shown
Required, but never shown
Required, but never shown
2RVBvQSfb1y,EdOA,gocrzhblFX