Linear Regression - Predict ŷ
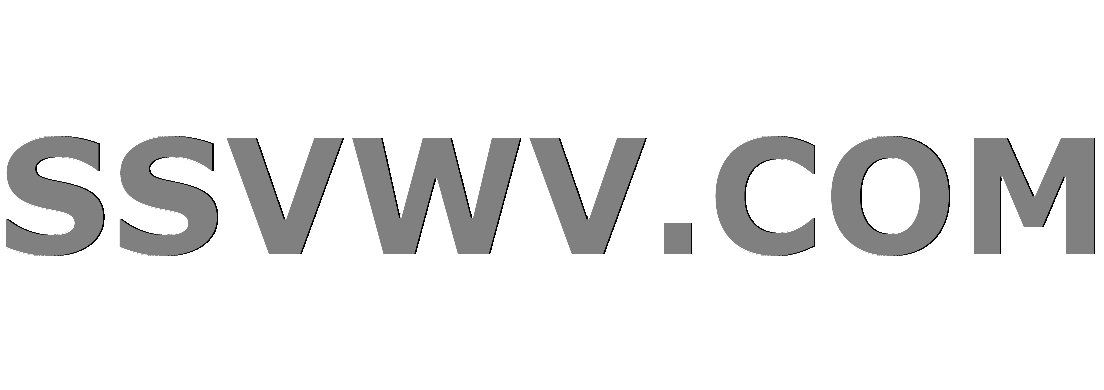
Multi tool use
up vote
-6
down vote
favorite
I'm trying to plot a scatter plot of the values of actual sales (y) and predicted sales (ŷ).
I have imported the csv file and currently the codes I have for the linear regression model is:
result = smf.ols('sales ~ discount + holiday + product', data=data).fit()
print(result.summary())
Since, I only have the actual sales values, how do I find the predicted sales (ŷ) values to plot the scatter plot? I have tried researching and found lm.predict()
and result.predict()
. Is there a difference? lm = LinearRegression()
Thank you in advance!
python linear-regression statsmodels predict
add a comment |
up vote
-6
down vote
favorite
I'm trying to plot a scatter plot of the values of actual sales (y) and predicted sales (ŷ).
I have imported the csv file and currently the codes I have for the linear regression model is:
result = smf.ols('sales ~ discount + holiday + product', data=data).fit()
print(result.summary())
Since, I only have the actual sales values, how do I find the predicted sales (ŷ) values to plot the scatter plot? I have tried researching and found lm.predict()
and result.predict()
. Is there a difference? lm = LinearRegression()
Thank you in advance!
python linear-regression statsmodels predict
Please clarify what you mean by ‚predicted sales‘. Why do you make a regression if you do not consider it to be the prediction?
– MisterMiyagi
Nov 10 at 10:30
Predicted sales based on all the x variables in the regression model so that I can plot the actual sales and predicted sales on a scatter plot.
– Smile
Nov 10 at 10:38
I dont really understand the downvotes here. You can get your predicted values by calling result.predict(), which will be your yhat values
– Simon
Nov 10 at 10:45
@Simon The question leaves it entirely unclear what problem there actually is. The problem itself is trivial and the two variants of ‚predict‘ are not qualified - it is pretty difficult to tell the difference without knowing what the things even are.
– MisterMiyagi
Nov 10 at 10:52
add a comment |
up vote
-6
down vote
favorite
up vote
-6
down vote
favorite
I'm trying to plot a scatter plot of the values of actual sales (y) and predicted sales (ŷ).
I have imported the csv file and currently the codes I have for the linear regression model is:
result = smf.ols('sales ~ discount + holiday + product', data=data).fit()
print(result.summary())
Since, I only have the actual sales values, how do I find the predicted sales (ŷ) values to plot the scatter plot? I have tried researching and found lm.predict()
and result.predict()
. Is there a difference? lm = LinearRegression()
Thank you in advance!
python linear-regression statsmodels predict
I'm trying to plot a scatter plot of the values of actual sales (y) and predicted sales (ŷ).
I have imported the csv file and currently the codes I have for the linear regression model is:
result = smf.ols('sales ~ discount + holiday + product', data=data).fit()
print(result.summary())
Since, I only have the actual sales values, how do I find the predicted sales (ŷ) values to plot the scatter plot? I have tried researching and found lm.predict()
and result.predict()
. Is there a difference? lm = LinearRegression()
Thank you in advance!
python linear-regression statsmodels predict
python linear-regression statsmodels predict
edited Nov 10 at 11:50
asked Nov 10 at 10:17
Smile
14
14
Please clarify what you mean by ‚predicted sales‘. Why do you make a regression if you do not consider it to be the prediction?
– MisterMiyagi
Nov 10 at 10:30
Predicted sales based on all the x variables in the regression model so that I can plot the actual sales and predicted sales on a scatter plot.
– Smile
Nov 10 at 10:38
I dont really understand the downvotes here. You can get your predicted values by calling result.predict(), which will be your yhat values
– Simon
Nov 10 at 10:45
@Simon The question leaves it entirely unclear what problem there actually is. The problem itself is trivial and the two variants of ‚predict‘ are not qualified - it is pretty difficult to tell the difference without knowing what the things even are.
– MisterMiyagi
Nov 10 at 10:52
add a comment |
Please clarify what you mean by ‚predicted sales‘. Why do you make a regression if you do not consider it to be the prediction?
– MisterMiyagi
Nov 10 at 10:30
Predicted sales based on all the x variables in the regression model so that I can plot the actual sales and predicted sales on a scatter plot.
– Smile
Nov 10 at 10:38
I dont really understand the downvotes here. You can get your predicted values by calling result.predict(), which will be your yhat values
– Simon
Nov 10 at 10:45
@Simon The question leaves it entirely unclear what problem there actually is. The problem itself is trivial and the two variants of ‚predict‘ are not qualified - it is pretty difficult to tell the difference without knowing what the things even are.
– MisterMiyagi
Nov 10 at 10:52
Please clarify what you mean by ‚predicted sales‘. Why do you make a regression if you do not consider it to be the prediction?
– MisterMiyagi
Nov 10 at 10:30
Please clarify what you mean by ‚predicted sales‘. Why do you make a regression if you do not consider it to be the prediction?
– MisterMiyagi
Nov 10 at 10:30
Predicted sales based on all the x variables in the regression model so that I can plot the actual sales and predicted sales on a scatter plot.
– Smile
Nov 10 at 10:38
Predicted sales based on all the x variables in the regression model so that I can plot the actual sales and predicted sales on a scatter plot.
– Smile
Nov 10 at 10:38
I dont really understand the downvotes here. You can get your predicted values by calling result.predict(), which will be your yhat values
– Simon
Nov 10 at 10:45
I dont really understand the downvotes here. You can get your predicted values by calling result.predict(), which will be your yhat values
– Simon
Nov 10 at 10:45
@Simon The question leaves it entirely unclear what problem there actually is. The problem itself is trivial and the two variants of ‚predict‘ are not qualified - it is pretty difficult to tell the difference without knowing what the things even are.
– MisterMiyagi
Nov 10 at 10:52
@Simon The question leaves it entirely unclear what problem there actually is. The problem itself is trivial and the two variants of ‚predict‘ are not qualified - it is pretty difficult to tell the difference without knowing what the things even are.
– MisterMiyagi
Nov 10 at 10:52
add a comment |
1 Answer
1
active
oldest
votes
up vote
0
down vote
Without data it is hard to help, but I guess you have X
and y
from dataset because you want to perform linear regression. You can split data into training and test set using scikit-learn
:
from sklearn.cross_validation import train_test_split
X_train, X_test, y_train, y_test = train_test_split(X, y, test_size = 1/3)
Then you need to fit linear regression to the training set:
from sklearn.linear_model import LinearRegression
regressor = LinearRegression()
regressor.fit(X_train, y_train)
and afterwards predict test set results:
y_pred = regressor.predict(X_test)
Finally, you can plot your test or training results:
# Visualising the Training set results
plt.scatter(X_train, y_train, color = 'red')
plt.plot(X_train, regressor.predict(X_train), color = 'blue')
plt.title('Discount vs Sales (Training set)')
plt.xlabel('Discount percentage')
plt.ylabel('Sales')
plt.show()
# Visualising the Test set results
plt.scatter(X_test, y_test, color = 'red')
plt.plot(X_train, regressor.predict(X_train), color = 'blue')
plt.title('Discount vs Sales (Test set)')
plt.xlabel('Discount percentage')
plt.ylabel('Sales')
plt.show()
(In this scenario we want to predict how many Sales will be if we set specific value of e.g. Discount percentage). If you have more than one X
parameter, things are more complicated and you will need to use dummy variables, perform statistical analysis etc..
add a comment |
1 Answer
1
active
oldest
votes
1 Answer
1
active
oldest
votes
active
oldest
votes
active
oldest
votes
up vote
0
down vote
Without data it is hard to help, but I guess you have X
and y
from dataset because you want to perform linear regression. You can split data into training and test set using scikit-learn
:
from sklearn.cross_validation import train_test_split
X_train, X_test, y_train, y_test = train_test_split(X, y, test_size = 1/3)
Then you need to fit linear regression to the training set:
from sklearn.linear_model import LinearRegression
regressor = LinearRegression()
regressor.fit(X_train, y_train)
and afterwards predict test set results:
y_pred = regressor.predict(X_test)
Finally, you can plot your test or training results:
# Visualising the Training set results
plt.scatter(X_train, y_train, color = 'red')
plt.plot(X_train, regressor.predict(X_train), color = 'blue')
plt.title('Discount vs Sales (Training set)')
plt.xlabel('Discount percentage')
plt.ylabel('Sales')
plt.show()
# Visualising the Test set results
plt.scatter(X_test, y_test, color = 'red')
plt.plot(X_train, regressor.predict(X_train), color = 'blue')
plt.title('Discount vs Sales (Test set)')
plt.xlabel('Discount percentage')
plt.ylabel('Sales')
plt.show()
(In this scenario we want to predict how many Sales will be if we set specific value of e.g. Discount percentage). If you have more than one X
parameter, things are more complicated and you will need to use dummy variables, perform statistical analysis etc..
add a comment |
up vote
0
down vote
Without data it is hard to help, but I guess you have X
and y
from dataset because you want to perform linear regression. You can split data into training and test set using scikit-learn
:
from sklearn.cross_validation import train_test_split
X_train, X_test, y_train, y_test = train_test_split(X, y, test_size = 1/3)
Then you need to fit linear regression to the training set:
from sklearn.linear_model import LinearRegression
regressor = LinearRegression()
regressor.fit(X_train, y_train)
and afterwards predict test set results:
y_pred = regressor.predict(X_test)
Finally, you can plot your test or training results:
# Visualising the Training set results
plt.scatter(X_train, y_train, color = 'red')
plt.plot(X_train, regressor.predict(X_train), color = 'blue')
plt.title('Discount vs Sales (Training set)')
plt.xlabel('Discount percentage')
plt.ylabel('Sales')
plt.show()
# Visualising the Test set results
plt.scatter(X_test, y_test, color = 'red')
plt.plot(X_train, regressor.predict(X_train), color = 'blue')
plt.title('Discount vs Sales (Test set)')
plt.xlabel('Discount percentage')
plt.ylabel('Sales')
plt.show()
(In this scenario we want to predict how many Sales will be if we set specific value of e.g. Discount percentage). If you have more than one X
parameter, things are more complicated and you will need to use dummy variables, perform statistical analysis etc..
add a comment |
up vote
0
down vote
up vote
0
down vote
Without data it is hard to help, but I guess you have X
and y
from dataset because you want to perform linear regression. You can split data into training and test set using scikit-learn
:
from sklearn.cross_validation import train_test_split
X_train, X_test, y_train, y_test = train_test_split(X, y, test_size = 1/3)
Then you need to fit linear regression to the training set:
from sklearn.linear_model import LinearRegression
regressor = LinearRegression()
regressor.fit(X_train, y_train)
and afterwards predict test set results:
y_pred = regressor.predict(X_test)
Finally, you can plot your test or training results:
# Visualising the Training set results
plt.scatter(X_train, y_train, color = 'red')
plt.plot(X_train, regressor.predict(X_train), color = 'blue')
plt.title('Discount vs Sales (Training set)')
plt.xlabel('Discount percentage')
plt.ylabel('Sales')
plt.show()
# Visualising the Test set results
plt.scatter(X_test, y_test, color = 'red')
plt.plot(X_train, regressor.predict(X_train), color = 'blue')
plt.title('Discount vs Sales (Test set)')
plt.xlabel('Discount percentage')
plt.ylabel('Sales')
plt.show()
(In this scenario we want to predict how many Sales will be if we set specific value of e.g. Discount percentage). If you have more than one X
parameter, things are more complicated and you will need to use dummy variables, perform statistical analysis etc..
Without data it is hard to help, but I guess you have X
and y
from dataset because you want to perform linear regression. You can split data into training and test set using scikit-learn
:
from sklearn.cross_validation import train_test_split
X_train, X_test, y_train, y_test = train_test_split(X, y, test_size = 1/3)
Then you need to fit linear regression to the training set:
from sklearn.linear_model import LinearRegression
regressor = LinearRegression()
regressor.fit(X_train, y_train)
and afterwards predict test set results:
y_pred = regressor.predict(X_test)
Finally, you can plot your test or training results:
# Visualising the Training set results
plt.scatter(X_train, y_train, color = 'red')
plt.plot(X_train, regressor.predict(X_train), color = 'blue')
plt.title('Discount vs Sales (Training set)')
plt.xlabel('Discount percentage')
plt.ylabel('Sales')
plt.show()
# Visualising the Test set results
plt.scatter(X_test, y_test, color = 'red')
plt.plot(X_train, regressor.predict(X_train), color = 'blue')
plt.title('Discount vs Sales (Test set)')
plt.xlabel('Discount percentage')
plt.ylabel('Sales')
plt.show()
(In this scenario we want to predict how many Sales will be if we set specific value of e.g. Discount percentage). If you have more than one X
parameter, things are more complicated and you will need to use dummy variables, perform statistical analysis etc..
answered Nov 10 at 12:42
Dejan Marić
436212
436212
add a comment |
add a comment |
Thanks for contributing an answer to Stack Overflow!
- Please be sure to answer the question. Provide details and share your research!
But avoid …
- Asking for help, clarification, or responding to other answers.
- Making statements based on opinion; back them up with references or personal experience.
To learn more, see our tips on writing great answers.
Some of your past answers have not been well-received, and you're in danger of being blocked from answering.
Please pay close attention to the following guidance:
- Please be sure to answer the question. Provide details and share your research!
But avoid …
- Asking for help, clarification, or responding to other answers.
- Making statements based on opinion; back them up with references or personal experience.
To learn more, see our tips on writing great answers.
Sign up or log in
StackExchange.ready(function ()
StackExchange.helpers.onClickDraftSave('#login-link');
);
Sign up using Google
Sign up using Facebook
Sign up using Email and Password
Post as a guest
Required, but never shown
StackExchange.ready(
function ()
StackExchange.openid.initPostLogin('.new-post-login', 'https%3a%2f%2fstackoverflow.com%2fquestions%2f53237954%2flinear-regression-predict-y%25cc%2582%23new-answer', 'question_page');
);
Post as a guest
Required, but never shown
Sign up or log in
StackExchange.ready(function ()
StackExchange.helpers.onClickDraftSave('#login-link');
);
Sign up using Google
Sign up using Facebook
Sign up using Email and Password
Post as a guest
Required, but never shown
Sign up or log in
StackExchange.ready(function ()
StackExchange.helpers.onClickDraftSave('#login-link');
);
Sign up using Google
Sign up using Facebook
Sign up using Email and Password
Post as a guest
Required, but never shown
Sign up or log in
StackExchange.ready(function ()
StackExchange.helpers.onClickDraftSave('#login-link');
);
Sign up using Google
Sign up using Facebook
Sign up using Email and Password
Sign up using Google
Sign up using Facebook
Sign up using Email and Password
Post as a guest
Required, but never shown
Required, but never shown
Required, but never shown
Required, but never shown
Required, but never shown
Required, but never shown
Required, but never shown
Required, but never shown
Required, but never shown
KlzBquLQB90SvGiTsNJRlR,B Fi yZM7S82,va7oqnVo,0e 6Kc LN1yXow,m3DOGM cSO0FouP4ybdlzcsvpdKpA3PV P0ptYgFAsh
Please clarify what you mean by ‚predicted sales‘. Why do you make a regression if you do not consider it to be the prediction?
– MisterMiyagi
Nov 10 at 10:30
Predicted sales based on all the x variables in the regression model so that I can plot the actual sales and predicted sales on a scatter plot.
– Smile
Nov 10 at 10:38
I dont really understand the downvotes here. You can get your predicted values by calling result.predict(), which will be your yhat values
– Simon
Nov 10 at 10:45
@Simon The question leaves it entirely unclear what problem there actually is. The problem itself is trivial and the two variants of ‚predict‘ are not qualified - it is pretty difficult to tell the difference without knowing what the things even are.
– MisterMiyagi
Nov 10 at 10:52